Stamatis P. Georgopoulos, Ph.D.
Nothing in life is to be feared, it is only to be understood. Now is the time to understand more, so that we may fear less. – Marie Curie
Nutrition Science: the complexities of nutrients and their health impacts
Nutrition science, the study of what we eat and how it affects us, is a relatively new scientific field. Starting with the discovery of the first vitamin less than 100 years ago, scientists documented and proved how certain diseases were prevented and treated by eliminating deficiencies of specific vitamins.
The successful prevention of single nutrient deficiency diseases led to nutrition research and policy recommendations to focus on single nutrients linked to specific disease states. However, single nutrient theories were inadequate to explain many effects of diet on non-communicable diseases.
More recently, scientific focus has shifted towards complex biological effects of foods and diet patterns to address the challenges for understanding the joint contributions and interactions of nutrients and how they influence health and wellbeing (Mozaffarian et al., 2018).
AI and the black box approach to getting answers to complex questions
I prefer ineloquent knowledge to loquacious ignorance. – Marcus Tullius Cicero
Artificial intelligence (AI) methods harness the knowledge in large datasets to provide solutions and recommendations on well-defined problems. Let us consider a simple example. You are sitting at a restaurant that serves only three items: potato chips, burgers, and soda drinks. You are able to see the quantities of each of those items a customer buys and the final price they pay. Let’s say we want a model
that given the quantities of each item, we get the final price.
Traditionally, we would solve this problem by providing the intelligence behind the solution. Our model would be this: Multiply each item by their price and add them up!
chips x price-chips + burgers x price-burgers + sodas x price-sodas = $$$
It is actually a quite easy problem for a mathematician; we need to solve a system of equations (our observations) with three unknowns (the prices of each item). Therefore if we observe enough different customers to get at least three equations we can solve for the item prices and then be able to predict the final price each time a new patron comes in.
AI methods do not do that.
What they usually do is, given the number of items as input, they start with a model with random components that will give a random final price prediction. This is initially wrong, but every time they get it wrong they slightly improve the model in order to nudge the prediction towards the correct answer. Rinse and repeat. Given enough observations the final AI model would correctly predict the final price for a new customer given the items they have picked. It will be indistinguishable from the traditional model in terms of results. The only difference is that we would not know how it did it. We open the black box, put in the number of items and out pops the final price.
And that is the beauty of it.
We never specified the hows and whats, we just gave the AI enough observations and it learned how to arrive at the correct answer on its own. In the real world, it is rare to have such a good understanding of
how a process works as we do in this example. Even in this case if, say, there is a 20% reduction after an order of more than $20, or every second burger in an order gets 50% off, our traditional model would be less accurate in its final predictions while the AI model would have learned those intricacies, simply by gradually improving every time it got something wrong.
AI models build a bridge from the data to the answer we are looking for without us having to specify the process behind it. That is why they have been really successful in fields such as medicine and drug discovery where the answer can be the result of a complex process with thousands of moving parts. That is why they are recently gaining traction as the go-to technology for nutrition R&D. We now have rich data and we are way past the point where the complexity can be handled by simple solutions. However, AI comes at the cost of not providing an answer as to how the magic works; the results are often opaque and in certain fields transparency is not only useful but in many cases a hard requirement.
Using explainable AI to build trust and increase understanding
The knowledge of anything, since all things have causes, is not acquired or complete unless it is known by its causes. – Abu Ali al- Hussain Ibn Abdallah Ibn Sina (Avicenna)
Explainable AI aims to provide an explanation or give justification behind each model decision. There are multiple benefits that explainability brings in the use of AI in nutrition science and arguably any science. Understanding how models arrive at a decision is crucial to building trust around their predictions. Furthermore, decision makers and experts can calibrate their trust on the outcomes given the basis of the suggested prediction. Interpretable results, in addition to being a safeguard against blatant errors, can also shorten the feedback loop and highlight areas for improvement. Transparency and interpretability also help stakeholders better understand the risks behind a decision they have to base on the AI output.
Google gives a fitting example of this about a route recommendations AI system (link):
“Be careful. It’s after 6pm and our route recommendations don’t include street light data.”
Transparently highlighting the model’s lack of data improves one’s ability to make a decision. Maybe the decision maker knows that the streets are well lit in the suggested route so they would be able to combine their knowledge and the AI recommendation to arrive at an optimal decision. They can safely ignore the “be careful” suggestion in this case because they know the reasoning behind it. The same suggestion with the same exact reasoning might lead to an entirely different decision for another user in an unfamiliar neighborhood if well-lit streets were important to them. In both situations if they had received a “be careful on your evening run” recommendation without any explanation they would have made a less informed decision.
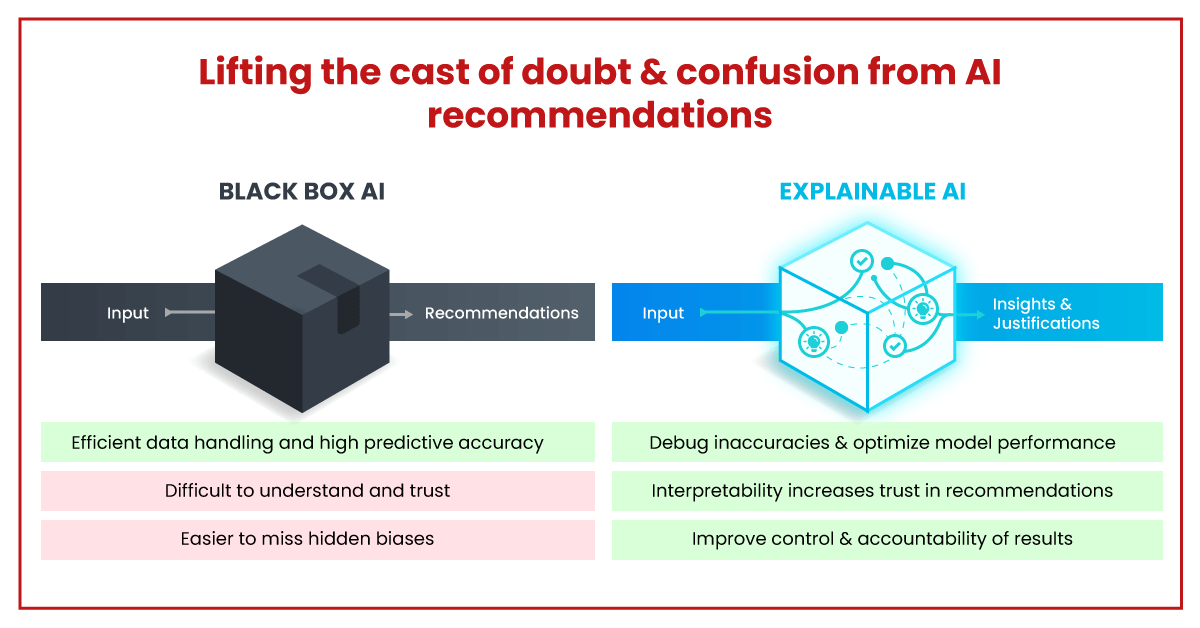
There are certainly challenges in achieving explainable AI. Some things may have very complex explanations, hard for humans or non experts, to really understand. Some explanations might have
valid but counterintuitive reasoning behind them. Other explanations might be too simplistic and give a less accurate picture than the AI sees by looking at a plethora of data and interactions. This might lead to situations where trade offs have to be made between accuracy and explainability which is always a tough decision to make, more so in the life sciences where a more powerful model might lead to a measurable impact on millions of peoples’ health and wellbeing.
Nature, and plants in particular, hold the key to unlocking a vast, still unchartered territory of bioactive compounds with potential health benefits for humans and animals alike. AI-enabled tools like LEAP™, aim to facilitate the discovery of promising, novel compounds by tapping into current and historical scientific
knowledge and using Machine Learning to predict new connections, correlations, and mediators.
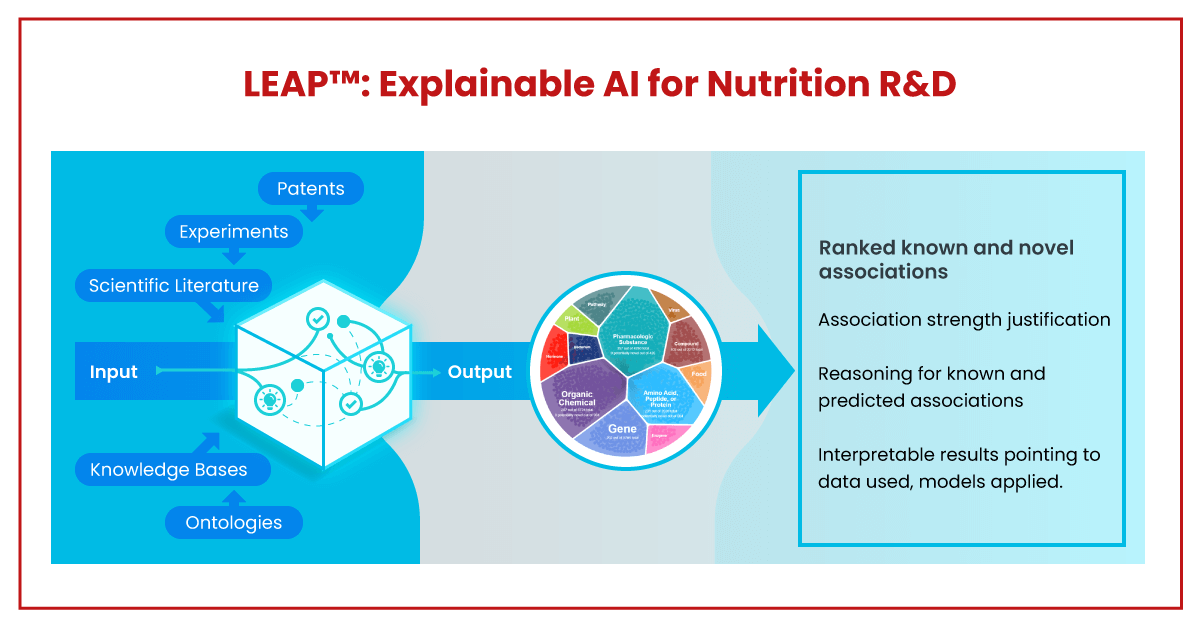
The challenges of tomorrow: Cracking open the black box of AI in nutrition
We choose to go to the Moon in this decade and do the other things, not because they are easy, but because they are hard, because that goal will serve to organize and measure the best of our energies and skills, because that challenge is one that we are willing to accept, one we are unwilling to postpone, and one which we intend to win, and the others, too. – John F. Kennedy
But difficulty and challenges are not standing in the way, when a goal is worth pursuing. Given the data rich environment we find ourselves in, in nutrition science, the complexity of the current scientific problems, the power of modern AI tools and the torch that explainable AI brings to the field we are at the cusp of major breakthroughs.
As we approach the 100 year mark since the discovery of the first vitamin I am willing to make a prediction; the era, explainable AI is going to usher in nutrition science, will be even more remarkable than the prevention of deficiency diseases a century ago.