The rapid growth of biomedical literature presents both an opportunity and a challenge for researchers and product developers in the nutrition and health industry. Buried within this vast trove of scientific publications are invaluable insights that could drive innovation and unlock unprecedented milestones. However, manual analysis and data extraction are prohibitively time-consuming, yet leveraging advanced algorithms experts can surface crucial connections that would be nearly impossible to detect unaided.
In our previous deep dive, we discussed the application of AI in biomedical data extraction. Take a moment to read it here. |
To enable scientific discovery, PIPA harnesses supervised relation extraction (RE) and open information extraction to elicit insights from vast data. Supervised RE, with its advanced machine learning techniques such as natural language processing (NLP) and large language models (LLMs), offers a powerful solution. It enables the extraction of meaningful relations between entities such as chemicals, foods, and diseases straight from texts, allowing for the annotation of large amounts of data with minimal human interaction.
Let’s dive into a practical, real-world scenario that demonstrates both the power of supervised RE and the capabilities of our AI-powered platform, LEAP™.
Technologies Used
To effectively train supervised RE models in the biomedical domain, we use LLMs, specifically transformer models pre-trained on biomedical corpora available in known online repositories. In doing so, we benefit from the abundance of domain-specific knowledge and linguistic patterns available in biological literature. If you are interested to learn more information on how we train these models, check out the first part of this blogpost series.
Highlighted Use Cases
LEAP, is an AI co-pilot that integrates scientific literature, vetted knowledge bases, and omics data to provide a unified, interconnected map of health and nutrition, augmented with novel insights and predictions. It empowers researchers and product developers to identify and investigate in-depth connections for over 870K+ entities and health conditions, analyze their relationship types in scholarly literature, collect evidence, and expedite bioactive breakthroughs.
We will present a use case in which we leverage LEAP and its supervised RE capability to identify positive correlations between ginger and medical conditions.
Ginger, treats, Disease
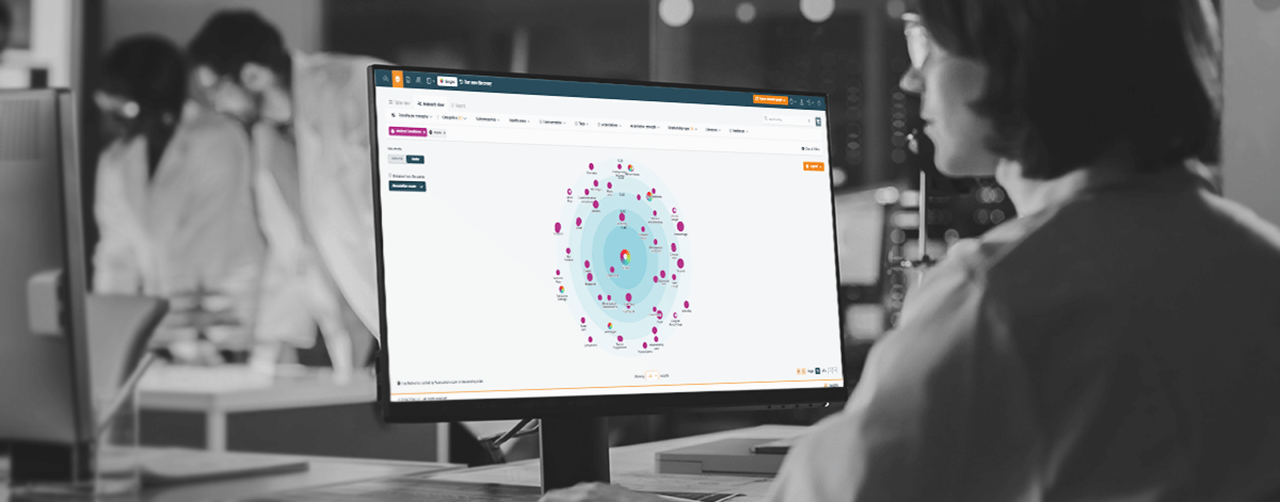
Through LEAP’s pipelines, ginger is associated with over 540 medical conditions. The question is: how can we identify the specific medical conditions that have a positive relationship type such as treats, prevents with ginger. This is where supervised RE comes into play; it identifies and classifies semantic relationships between entities within the scientific literature. Powered by supervised RE, LEAP enables researchers to readily identify over 270 medical conditions that are reported to be treated or prevented by ginger.
Every relationship type comes with its number of related articles, based on which users can estimate the quantity of studies conducted and prioritize results accordingly. In LEAP, some of the top-ranked conditions that ginger is commonly used to treat include nausea (11 articles), diarrhea (19 articles), and osteoarthritis (23 articles), which may be more valuable than conditions such as type 2 diabetes that has only 2 associated articles.
Evaluation of Performance
The supervised RE models used in our products implement specific standards. They extract a plethora of triplets achieving high precision and recall, which are defined as follows:
- Precision means that when our system identifies a relation between biomedical entities (such as food and disease), how often that relation is correctly identified. High precision means the system provides highly accurate triplets, reducing the risk of false or incorrect information.
- Recall indicates the system’s ability to find all relevant triplets within the data. High recall means that the system misses very few actual triplets, which is crucial for comprehensive data analysis and ensuring no valuable insights are overlooked.
Both precision and recall are important because in the medical field, missing out on critical information (low recall) or getting incorrect information (low precision) pose significant challenges.
Moving forward
As the field of artificial intelligence continues to advance, the potential of biomedical supervised RE is poised to grow exponentially. With ongoing improvements in natural language processing, knowledge representation, and machine learning algorithms, this technology is expected to become increasingly accurate, scalable, and versatile. As we look to the future, PIPA is committed to continue empowering research teams and product developers to make faster, more informed decisions while significantly reducing resource expenditure for a more connected, sustainable, and intelligent world.